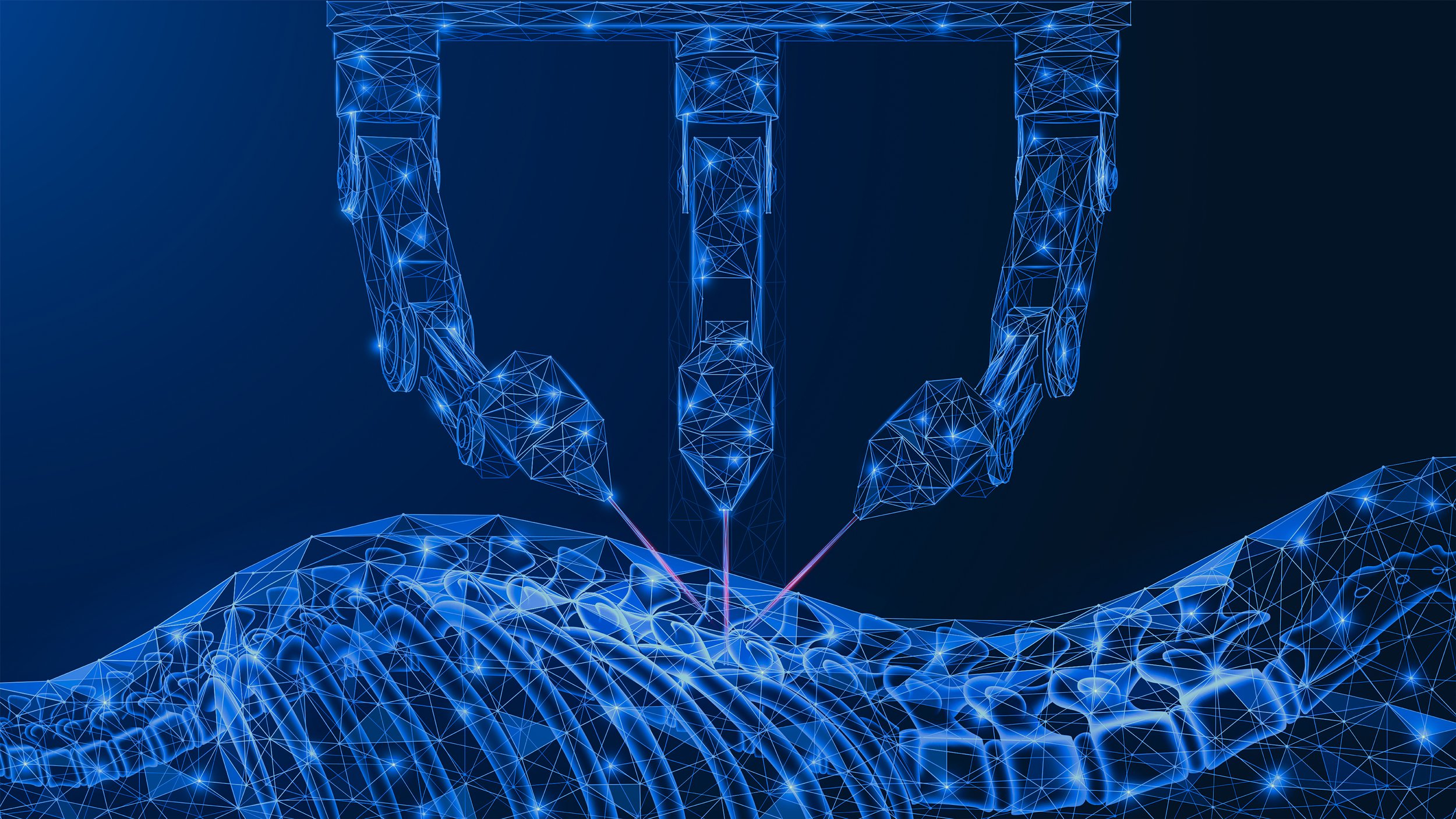
AI
AI and Surgical Metrics
Ultimate surgical training goals are to shorten learning curves, improve cognitive decision making, gain technical skills through simulation and deliberate practice, and provide real-time and ongoing feedback that will drive effective performance of operations with the best possible outcomes for patients. There is a growing body of evidence demonstrating that artificial intelligence (AI) and machine learning (ML) are positioned to play a pivotal role in the future of surgical education and training. These technologies enable analysis of data from multi-modal sensors on cognitive, technical, physiological, and functional correlates of surgical performance.
ISE convened, hosted, and facilitated two AI and Surgical Metrics consensus conferences attended by a broad range of stakeholders, including surgeons, educators, engineers, computer scientists, experts affiliated with professional surgical societies, and regulatory agencies. Through the Delphi process, the conference:
Achieved consensus on the utility, anticipated key applications, and challenges in translating AI and metrics for surgical education.
Addressed how AI can be close the loop for surgical performance assessment and feedback between simulation and the operating room (OR).
Produced a roadmap and action plan for researchers in the field of AI in surgery to follow for the next decade, which is described below.
Short-term
(2-year timeframe)
01. Automated recognition of instruments and their movements, anatomical structures, procedural steps, and errors in surgical videos
02. Optimize and standardize surgical video annotation
03. Automated performance assessment and augmented feedback (formative and summative) for trainees
Mid-term
(5-year timeframe)
04. Identify parts of the operation on which the surgeon needs feedback
05. Overlay images to display surrounding anatomy
06. Guide surgeons on exposure of the surgical field
07. Guide surgeons on optimal use of instruments
Long-term
(10-year timeframe)
08. Enable intraoperative navigability using video, kinematics, and other imaging data for multiple procedures
09. Detect intraoperative errors
10. Provide guidance on the next best step to address an intraoperative error or complication
To support this action plan, ISE formed a Collaborative for Advanced Assessment of Robotic Surgical Skills (CAARSS), which is described in AI Research Group (CAARSS) section of the website.